


30


25
20
15
10
5
0
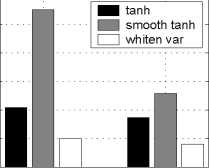
179-rule predictive rule
(b)
(a)
Fig. 3. a) Spectrograms of some of the sources separated using variance whitening.
Time on the horizontal and frequency on the vertical axis. b) Used processing time for
different denoising functions and step sizes.
on the denoising function. Tanh-nonlinearity with smoothed variance estimate
used a fixed spectral shift and benefitted more from adaptation of γ than the
plain tanh-nonlinearity with FastICA-type spectral shift.
6 Conclusion
DSS framework offers a sound basis for developing simple but efficient and accu-
rate source separation algorithms. We proposed a method for stabilising and ac-
celerating convergence and showed that convergence is faster than with FastICA.
Additional benefit is that gradient of the nonlinearity is not needed. We also
proposed a new denoising procedure which was justified as the MAP-estimate of
signals with changing variance. Denoising which makes use of non-stationarity
of variance was shown to yield better results than the popular tanh-nonlinearity
as measured by SNR in the artificially generated data. The variance-whitening
DSS also extracted cleaner signals in MEG data, while the tanh-nonlinearity
had difficulties with some weak but clear phenomena. Whitening the estimated
variances of different sources significantly improved convergence.
References
1. Sareia, J., Valpola, H.: Denoising source separation. Submitted to a journal (2004)
Available at Cogprints http://cogprints.ecs.soton.ac.uk/archive/00003493/.
2. Hyvaarinen, A.: Fast and robust fixed-point algorithms for independent component
analysis. IEEE Trans. on Neural Networks 10 (1999) 626-634
3. Matsuoka, K., Ohya, M., Kawamoto, M.: A neural net for blind separation of
nonstationary signals. Neural Networks 8 (1995) 411-419
4. Schwartz, O., Simoncelli, E.P.: Natural signal statistics and sensory gain control.
Nature Neuroscience 4 (2001) 819 - 825
5. Cardoso, J.F.: High-order contrasts for independent component analysis. Neural
computation 11 (1999) 157 - 192
6. Sarela, J., Valpola, H., Vigario, R., Oja, E.: Dynamical factor analysis of rhythmic
magnetoencephalographic activity. In: Proc. Int. Conf. on Independent Component
Analysis and Signal Separation (ICA2001), San Diego, USA (2001) 451-456
More intriguing information
1. The name is absent2. The name is absent
3. Foreign direct investment in the Indian telecommunications sector
4. fMRI Investigation of Cortical and Subcortical Networks in the Learning of Abstract and Effector-Specific Representations of Motor Sequences
5. Political Rents, Promotion Incentives, and Support for a Non-Democratic Regime
6. Portuguese Women in Science and Technology (S&T): Some Gender Features Behind MSc. and PhD. Achievement
7. WP 36 - Women's Preferences or Delineated Policies? The development or part-time work in the Netherlands, Germany and the United Kingdom
8. Ex post analysis of the regional impacts of major infrastructure: the Channel Tunnel 10 years on.
9. The name is absent
10. The name is absent