(b) (c) (d)
(e)
(f)
Figure 1.3 : Two-dimensional comparison of methods, (a) is a multi-material voxel
with no intensity information, (b) is the naive approach of classifying by nearest
neighbor, (c) Tiede et al. proposes a linear filter for classification [27], but it leaves
points unclassified, (d) We propose a tri-linear representation that classifies all points
within a voxel, (e) and (f) are examples of our approach that demonstrate the flexi-
bility in representing contours.
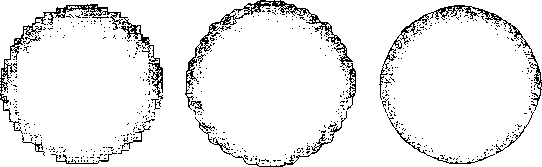
(a)
(c)
Figure 1.4 : Three-dimensional comparison of methods, (a) is the input of a sphere-
like segment, (b) is rendered using Tiede et al.’s classification scheme [27]. Note
that it produces a bumpy surface, (c) is our representation for the segment contour.
Details for constructing (c) from the segment (a) is described in Section 3.4.
volume rendering [8]. The classification scheme described by Tiede et al. focuses on
segments produced by thresholding. In the case where the threshold ranges of mul-
tiple materials overlap, Tiede et al.’s approach is to linearly interpolate the binary
mask associated with the material. For each material A, space where the interpolated
value (with respect to A’s tri-linearly interpolated binary mask) is greater than 0.5
is classified as A. Although linear filtering resolves the overlap of threshold ranges, it
also produces unclassified regions within a single voxel (see Figure 1.3(c)). In the case
where the input is a segmented volume without intensity information, Tiede et al’s
More intriguing information
1. Design and investigation of scalable multicast recursive protocols for wired and wireless ad hoc networks2. Luce Irigaray and divine matter
3. Pursuit of Competitive Advantages for Entrepreneurship: Development of Enterprise as a Learning Organization. International and Russian Experience
4. Transfer from primary school to secondary school
5. Credit Markets and the Propagation of Monetary Policy Shocks
6. Placenta ingestion by rats enhances y- and n-opioid antinociception, but suppresses A-opioid antinociception
7. The economic doctrines in the wine trade and wine production sectors: the case of Bastiat and the Port wine sector: 1850-1908
8. Testing for One-Factor Models versus Stochastic Volatility Models
9. The open method of co-ordination: Some remarks regarding old-age security within an enlarged European Union
10. Two-Part Tax Controls for Forest Density and Rotation Time