where Ib is the set of households in the bth block, and where Nb,1 and Nb,0 are the subsets within
Ib that fall either into the treatment group or control group. To get the average treatment effect
by the method of stratification, we simply weight each of these block-specific treatment effects
by the proportion of treated households falling into each block, and then sum the resulting
weighted block-specific treatment effects over all strata Thus,
6
i∈ibD Di
P Di
ATTStrat = X ATTb ×
b=1
4.2 Nearest-Neighbor Matching
One very attractive feature of matching on the propensity score is that we need not assume a
specific functional form for the underlying distribution of the treatment effect since the (average)
treatment effect can be computed semi-parametrically.
One such approach is to match each treated household to the control household that most
closely resembles it. There are various ways in which this can be done, one of which is to match
directly on x, but given Lemma 1, a better way to proceed is to match on the propensity score.
Since p(x) is a scalar index, this method has the advantage of permitting a greater number of
matches than matching directly on x would allow.
Formally, we can define the set of potential control group matches (based on the propensity
score) for the ith household in the treatment group with characteristics xi as
Ai(p(x)) = {pj | min |pi -pj|}
j
The matching set will usually contain more than one control group household that could po-
tentially feature in the calculation of the average treatment effect. The most restrictive form of
the nearest neighbor method would select a unique control group household for every treatment
group household on the basis of computing the absolute value of the difference in propensity
scores for every pairwise match considered, and then selecting as a match the jth household
with the smallest absolute difference in propensity scores. Alternatively, all observations in the
set Ai(p(x)) could be matched against household i. In this case, a differential weight would be
applied to each match falling into the matching set. The average treatment effect would then
be computed as follows:
ATTNN = (N1)-1 X (y1i-Σjω(i,j)y0j)
i∈{T =1}
where j is an element of Ai(p(x)) and ω(i, j) is the weight given to j. For the restrictive
one-to-one match mentioned above, we would then have ω(i, j) = 1 when j ∈ Ai (p(x)), and
ω(i, j) = 0 when j 3 Ai (p(x)).
4.3 Kernel Matching
A closely related approach to nearest-neighbour matching is to match non-parametrically using
a kernel function. In this instance our formula for the ATT is as above, but the weight given
to the jth control group household in matching it to the ith treated household is determined
as follows
ω(i,j) = Kx) - p(xi))
PN=1 K(p(Xj) - p(Xi))
K=
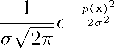
where K is the Gaussian (normal) kernel. This method has the benefit of using the entire
sample for each prediction with decreasing weights for more distant observations, where the
rate of decline of these weights is determined by σ . In principle, ω could be determined in other
ways (e.g., tri-cubic, caliper etc.)
More intriguing information
1. The name is absent2. The Impact of EU Accession in Romania: An Analysis of Regional Development Policy Effects by a Multiregional I-O Model
3. Partner Selection Criteria in Strategic Alliances When to Ally with Weak Partners
4. Emissions Trading, Electricity Industry Restructuring and Investment in Pollution Abatement
5. The name is absent
6. How does an infant acquire the ability of joint attention?: A Constructive Approach
7. The name is absent
8. The name is absent
9. Moffett and rhetoric
10. PERFORMANCE PREMISES FOR HUMAN RESOURCES FROM PUBLIC HEALTH ORGANIZATIONS IN ROMANIA