with a single summation unit, denoted by the symbol Σ . The collective outputs of these
modules constitute the input to the second processing layer consisting of J output units
that perform the flow prediction by applying some sort of the Bradley-Terry-Luce
model and enforcing satisfactorily the conservation rule of interaction from the
viewpoint of origins [destinations]4.
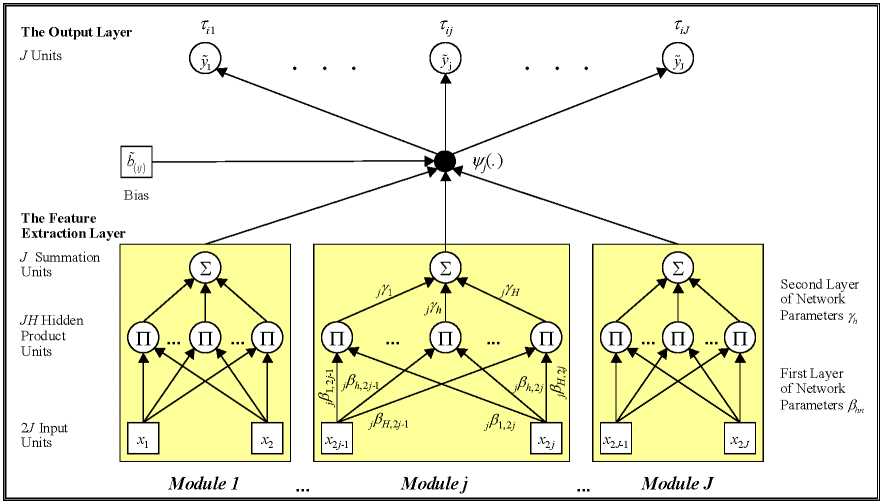
Figure 1: Architecture of the Product Unit Neural Spatial Interaction Model: The
Singly Constrained Case
The first layer of network parameters includes 2JH connection weights, so that
1 w ( j ^( j-1) H +1,2 j -1’ ..., j ^( j-1) H+h ,2 j-1’ "ɔ j PjH ,2 j-1, j P( j -1) H +1,2 j ’ ''" j P( j -1) H+h ,2 j ’ ''" j PjH ,2 j )
(10)
while the second layer contains JH weights:
2 w ( j ^( j-1) H +1’ ..., j ^( j-1) H+h’ ..., j ^ jH )
(11)
We have incorporated the basic trick of weight sharing into our network design to
reduce model complexity. Weight sharing involves forcing the set of connection
weights to be identical across the Jmodules. Thus, w = ( 1 w, 2 w) is a (3H)-dimensional
11
More intriguing information
1. The name is absent2. Regional science policy and the growth of knowledge megacentres in bioscience clusters
3. The name is absent
4. Creating a 2000 IES-LFS Database in Stata
5. Demographic Features, Beliefs And Socio-Psychological Impact Of Acne Vulgaris Among Its Sufferers In Two Towns In Nigeria
6. The name is absent
7. The name is absent
8. Human Development and Regional Disparities in Iran:A Policy Model
9. Technological progress, organizational change and the size of the Human Resources Department
10. The name is absent