324
Z Funke
variables with Eigendynamik control of the system turned out to be much
harder. This points to the fact that knowledge acquisition and knowledge
application require different abilities, which under certain circumstances lead
to a dissociation of both measures.
Experiment 3: Identification of Side Effects
Independent and dependent variables. In this third experiment, the effect of
three different degrees of side effects was analyzed. Side effects play a major
role in the complexity of system identification and control because in most
cases the side effects cannot be observed directly, but only via other
indicators. At the same time, side effects have -by definition- smaller effects
on the system variables than the strong main effects, thus making it more
difficult to detect their existence and their subtle influences on the other
system variables.
Side effects were operationalized as minor effects from one endogenous
variableon to another. In this case, parameters aaπd d from Fig. 1 and Eq. (2)
to (4) remained unchanged (1 resp. 0.9), but parameters b and c Werechanged
in three steps: b=0, c=0: a control condition without side effects (Condition
O); b=0, c=0.2: one side effect (Condition I); b=0,5, c=0.2: two side effects
(Condition 2). Dependent variables were again QSC as a measure for control
performance and QSI as indicator for acquired and verbalizable knowledge.
Subjects, material, and procedure. Under each of the three conditions eight
male subjects (Bonn University students) were run individually. According to
Cohen (1977), assuming α=0.10 and “large effects” (f=0.40) power l*β
proves to be 0.47 for the main effect. The system used was again SINUS with
the changes described above and with the following change of the procedure:
During the first four blocks exploration was not limited by number of trialbut
by time (15 min per block). During the fifth block all subjects were required
to reach and maintain the previously specified goal states over seven trials
without time pressure.
Results. The expected influence of side effects on knowledge acquisition was
confirmed by a significant negative path coefficient (β=-0.35, p ≤ 0.10) from
the side effect predictor to QSl (mean QSI for 0,1, and 2 side effects are 1.14,
1.26, and 0.77, F<wιf=l .74, n.s., respectively; see Fig. 3). Also, the effect from
knowledge onto control quality reached significance (β=0.73, p ≤ 0.10 from
QSI to QSC; mean QSC for 0, 1, and 2 side effects are 2.39,2.86, and 4.72,
Fc,11)=4.01, p ≤ 0.10, respectively; see Fig. 3). The number of trials in blocks
Chapter 14 Microworids Based on Linear Equation Systems
325
1 to 4 had (contrary to our expectation) no predictive value for QSC or QSI,
but this conclusion is taken only as preliminary because of medium power.
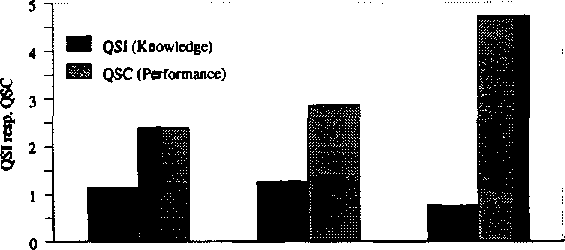
NumbBr of Side Effects
Figure 3 Number of Side Effects and its influence on the Vcrbalizable knowledge
(QSI: larger values indicating more knowledge) and control performance
(QSC; larger values indicating less control).
Discussion. As in the two previous experiments, the manipulation of another
system attribute shows an effect on knowledge acquisition as revealed by the
QSI measure and, again, the amount of knowledge predicts the quality of
system control QSC. This result is in line with Conant and Ashby (1970)
according to which good control has to be the consequence of a good model.
Discussion
This section deals first with a summarizing discussion of the experimental
results. The second part is concerned with a discussion of the DYNAMIS
approach as a general approach to study complex problem solving.
More intriguing information
1. The name is absent2. The name is absent
3. he Effect of Phosphorylation on the Electron Capture Dissociation of Peptide Ions
4. Explaining Growth in Dutch Agriculture: Prices, Public R&D, and Technological Change
5. Peer Reviewed, Open Access, Free
6. Technological progress, organizational change and the size of the Human Resources Department
7. A COMPARATIVE STUDY OF ALTERNATIVE ECONOMETRIC PACKAGES: AN APPLICATION TO ITALIAN DEPOSIT INTEREST RATES
8. Types of Cost in Inductive Concept Learning
9. Accurate and robust image superresolution by neural processing of local image representations
10. The name is absent