first factor determining the early clusters is the
Appropriateness of objects for the protection function.
Secondly, the clusters are then subdivided according to the
type of falling objects. Thirdly, scenes group into clusters
that have similar dimensions or shape components. Figure 4
shows a cluster diagram for this condition. A major
difference between this condition and the other two is that
up to the last level of clustering the appropriate and
inappropriate objects are always kept separate. In networks
A and B only at the level of the final clusters the two
objects are separated. Finally, one cluster out of five uses an
unclear and inconsistent grouping strategy.
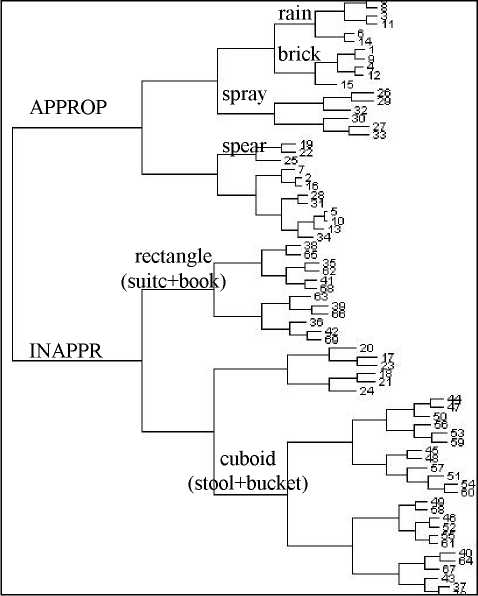
Figure 4: Cluster analysis diagram of the hidden units’ activation
of a network of condition C (feature-based encoding). Input
stimuli first group according to their function (i.e.,
approriateness/inappropriateness), and subsequently according to
the type of falling objects and the similarity of the shape
components. Pure geometrical factors such as the orientation of
the protecting object are ignored in the early stages of processing.
Overall, the results of hidden activation clustering show
that with architectures using localist encodings (networks A
and B), geometrical factors such as the orientation of the
protecting object prevail. When an explicit encoding of
extra-geometrical factors is used, as with architecture C, the
stimuli tend to primarily group according to variables
related to the function of objects. Most of these extra-
geometrical variables, such as the object’s lexical functional
appropriateness and its size, have been proven to greatly
affect the use and comprehension of spatial terms [2].
Therefore, the explicit encoding of objects’ extra-
geometrical properties (e.g. through feature-based input unit
of network C) and its subsequent effect on the network
processing strategies seem to more adequately reflect the
phenomena observed in experimental subjects. This better
match between the network and experimental data favors
the use of such a type of architecture for the further
development of a computational model of spatial language
and cognition.
4 Conclusion
This hybrid NN and VR system allowed us to model the
effects of functional and geometrical factors on the
comprehension of spatial prepositions. Moreover, it
provides a prototype NLP interface for interactive VR
applications.
Further research is being conducted in order to develop a
psychologically plausible neural network model for the
processing of spatial language. The current prototype model
shows the importance of explicitly encoding and inputting
the extra-geometrical features of objects, as well as their
geometrical properties. However, the use of a pre-defined
set of functional features and its distributed and explicit
encoding in the input units is not yet satisfactory. A
computational model of spatial language and cognition
should be able to derive, on-demand, and use the right set of
properties that are salient to the scene and its context. This
is the direction that we are following in our on-going
research.
References
[1] Carlson-Radvansky, L.A. & Radvansky, G.A. (1996). The
influence of functional relations on spatial term selection.
Psychological Science, 7(1), 56-60.
[2] Coventry, K.R. (in submission). Spatial prepositions and the
instantiation of object knowledge: the case of ‘over’, ‘under’,
‘above’ and ‘below’.
[3] Coventry, K.R. (1998). Spatial prepositions, functional
relations and lexical specification. In P. Olivier and K. Gapp
(Eds.), The Representation and Processing of Spatial Expressions,
pp247-262. Lawrence Erlbaum Associates.
[5] Coventry. K.R., Carmichael, R. & Garrod, S.C. (1994). Spatial
prepositions, functional relations and task requirements. Journal of
Semantics, 11, 289-309.
[4] Coventry, K.R., Prat-Sala, M. & Richards, L. (2001). The
interplay between geometry and function in the comprehension of
‘over’, ‘under’, ‘above’ and ‘below’. Journal of Memory and
Language, 44, 376-398.
[6] Feldman J., Fanty M. & Goddard N. (1988). Computing with
structured neural networks. IEEE Computer, 21, 91-104.
[7] Garrod, S.C. & Sanford, A.J. (1989). Discourse models as
interfaces between language and the spatial world. Journal of
Semantics, 6, 147-160.
More intriguing information
1. The name is absent2. MANAGEMENT PRACTICES ON VIRGINIA DAIRY FARMS
3. Rent-Seeking in Noxious Weed Regulations: Evidence from US States
4. The name is absent
5. Kharaj and land proprietary right in the sixteenth century: An example of law and economics
6. A MARKOVIAN APPROXIMATED SOLUTION TO A PORTFOLIO MANAGEMENT PROBLEM
7. AJAE Appendix: Willingness to Pay Versus Expected Consumption Value in Vickrey Auctions for New Experience Goods
8. The name is absent
9. The name is absent
10. Regional differentiation in the Russian federation: A cluster-based typification