stories and tales for children (~1,6 million words), children's
productions (~800,000 words), reading textbooks (~400,000
words) and children's encyclopedia (~400,000 words). This
corpus is composed of 57,878 paragraphs for a total of 3.2
million word occurrences. All punctuation signs were ruled
out, capital letters were transformed to lower cases, dashes
were ruled out except when forming a composed word (like
tire-bouchon). This corpus was analyzed by means of LSA
and the occurrence matrix reduced to 400 dimensions,
which appears to be an optimal value as we will see later.
The resulting semantic space contains 40,588 different
words. This step took 15 minutes on a 2.4 Ghz computer
with 2 Gb RAM.
Tests
In order to test whether this semantic space can be an
acceptable approximation of the semantic memory of
children, we tested three features: its extent, its organization
and its use. For each one, we relied on a specific task and
compared the data from the simulation of the task to data
obtained from children on the exact same task.
The extent feature has to do with the size of lexical
knowledge. Does our semantic space knows the kind of
words that a child knows? We used a vocabulary task for
that: given a word, the goal is to find the correct definition
from four of them. By comparing the model data with
children's data at various ages, our goal is to approximately
identify the kind of children we are mimicking.
The organization feature concerns the way words are
associated to others in memory. Do we correctly mimic the
semantic neighborhood of words? The task we used for
testing that feature is an association task :given a word, the
goal is to provide the most associated one. We will compare
children's association norms to association measures in the
semantic space.
The use feature has to do with the way semantic memory
is used. Is our semantic space adequate enough so that it can
account for a process that uses it? We used a recall task for
studying the text comprehension process which obviously
largely relies on semantic representations.
These three experiments cover different tasks and
different grain sizes of language entities, from words to
texts: the first one consists of word comparisons, the second
one compares a word and a sentence and the third one
compares texts. We expect a good match between human
data and model data. In addition, we hypothesize that results
will be higher with our children corpus than with adult
corpora.
Experiment 1
The first experiment, which aims at validating the model,
involves a vocabulary task. The design of the material as
well as the experiments with children were realized by
Denhiere et al. (in preparation). Material consists of 120
questions, each one composed of a word and four
definitions: the correct one, a close definition, a far
definition and an unrelated definition. For instance, given
the word nourriture (food), translations of the four
definitions are:
- what is used to feed the body (correct);
- what can be eaten (close);
- matter which is being spoiled (far);
- letter exchange (unrelated).
Participants were asked to select what they thought was the
correct definition. This task was performed by four groups
of children: 2nd grade, 3rd grade, 4th grade and 5th grade.
These data were compared with the cosines between the
given word and each of the four definitions. For instance,
the four cosines on the previous examples were: .38
(correct), .24 (close), .16 (far) and .04 (unrelated). 116
questions were used because the semantic space did not
contain four rare words.
The first measure we used was the percentage of correct
answers. Figure 1 displays the results. The percentage of
correct answers is .53 for the model, which is exactly the
same value as the 2nd grade children. Except for unrelated
answers, the model data globally follow the same pattern as
the children's data.
70
65
60
55
50
45
40
35
30
25
20
15
10
5
0
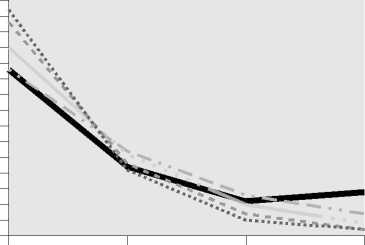
Close
Far
Definition types

Correct
Unrelated
Figure 1: Percentage of answers for different types of
definitions
In order to compare our semantic spaces with adult semantic
spaces, we defined a measure which integrates the four
values. We used a d measure, which is a normalized
difference between the cosines for correct and close
definitions together and the cosines for far and unrelated
definitions together. The higher this measure, the better the
result. Given a word W, four definitions (correct, close, far
and unrelated) and a global standard deviation S, the
formula is the following:
cos (W, correct)+cos (W, close cos (W, far)+cos (W, unrelated)
d=
We also compared these results with several adult corpora,
in order to test whether our semantic space was specific to
children. We used five corpora: a literature corpus,
composed of novels from the XIXth and XXth centuries and
four corpora from the French daily newspaper Le Monde, of
the years 1993, 1995, 1997 and 1999. Table 1 shows the
results.
More intriguing information
1. A Hybrid Neural Network and Virtual Reality System for Spatial Language Processing2. Accurate and robust image superresolution by neural processing of local image representations
3. The name is absent
4. Bargaining Power and Equilibrium Consumption
5. Yield curve analysis
6. Demographic Features, Beliefs And Socio-Psychological Impact Of Acne Vulgaris Among Its Sufferers In Two Towns In Nigeria
7. What Contribution Can Residential Field Courses Make to the Education of 11-14 Year-olds?
8. The name is absent
9. ANTI-COMPETITIVE FINANCIAL CONTRACTING: THE DESIGN OF FINANCIAL CLAIMS.
10. Wounds and reinscriptions: schools, sexualities and performative subjects