1.5 Estimation of parameters
11
Sample of size N=10^4
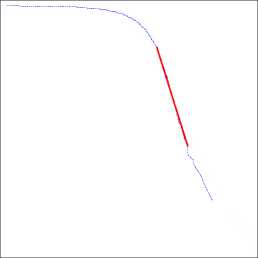
-4 -2 0 2 4
log(x)
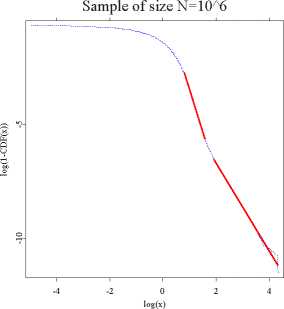
Figure 1.4: A double logarithmic plot of the right tail of an empirical symmetric
1.9-stable distribution function for a sample of size N = 104 (left
panel) and N = 106 (right panel). Thick red lines represent the
linear regression fit. The tail index estimate (<^ = 3.7320) obtained
for the smaller sample is close to the initial power-law like decay of
the larger sample (<^ = 3.7881). The far tail estimate α = 1.9309 is
close to the true value of α.
θ STFstab04.xpl
i.e. the negative tail) observations, after a crossover from a temporary power-
like decay (which surprisingly indicates α ≈ 3.7). Moreover, the obtained
estimates still have a slight positive bias, which suggests that perhaps even
larger samples than 106 observations should be used. In Figure 1.4 we used
only the upper 0.15% of the records to estimate the true tail exponent. In
general, the choice of the observations used in the regression is subjective and
can yield large estimation errors, a fact which is often neglected in the literature.
A well known method for estimating the tail index that does not assume a
parametric form for the entire distribution function, but focuses only on the
tail behavior was proposed by Hill (1975). The Hill estimator is used to estimate
the tail index α, when the upper (or lower) tail of the distribution is of the
form: 1 - F (x) = Cx-α, see Figure 1.5. Like the log-log regression method, the
Hill estimator tends to overestimate the tail exponent of the stable distribution
More intriguing information
1. 5th and 8th grade pupils’ and teachers’ perceptions of the relationships between teaching methods, classroom ethos, and positive affective attitudes towards learning mathematics in Japan2. School Effectiveness in Developing Countries - A Summary of the Research Evidence
3. Quality practices, priorities and performance: an international study
4. Educational Inequalities Among School Leavers in Ireland 1979-1994
5. The name is absent
6. Modellgestützte Politikberatung im Naturschutz: Zur „optimalen“ Flächennutzung in der Agrarlandschaft des Biosphärenreservates „Mittlere Elbe“
7. Bird’s Eye View to Indonesian Mass Conflict Revisiting the Fact of Self-Organized Criticality
8. Cross-Country Evidence on the Link between the Level of Infrastructure and Capital Inflows
9. CONSUMER ACCEPTANCE OF GENETICALLY MODIFIED FOODS
10. Disentangling the Sources of Pro-social Behavior in the Workplace: A Field Experiment