16
Stata Technical Bulletin
STB-4
better mental health merely tend to be married (manifestation). Our results are consistent with the manifestation hypothesis (the
estimated coefficient is negative) but they do not exclude a positive coefficient because of the insignificance of the measured
effect.
The age effect, however, is more difficult to disentangle because the patients age over time. Note that the average slope
is positive, which means that most people in the sample have increasing mental health over time or, said differently, as they
age. This supports a causal hypothesis over a cohort difference hypothesis. The age coefficient, on the other hand, is negative,
so the impact of increasing mental health with age must dampen with age. That was consistent with the cross-sectional results,
even though they were not significant!
There is an important point here. Let’s pretend that our data had been “clean” in that there were no missing values and
repeated measures ANOVA had been a real alternative. One should feel uncomfortable performing a repeated measures ANOVA
analysis at the outset. For instance, there was no guarantee that the age effects we found would be consistent and, had they not
been, repeated measures ANOVA would have oversimplified the problem. If one has complete data, repeated measures ANOVA
can well be a convenient summary device after one has verified the assumptions using the techniques of the sort outlined above.
And if one does not have complete data, repeated measures ANOVA is an even less attractive alternative.
The techniques outlined above are hardly a definitive treatise on the analysis of this sort of data. I invite readers to comment
on how they would analyze such data and we will follow up in a later issue.
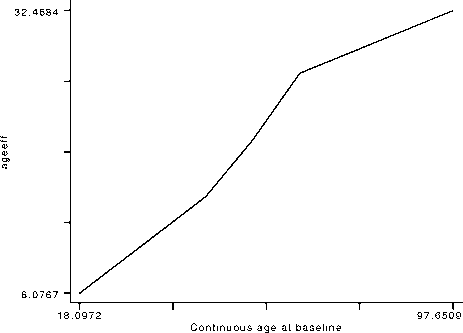
Figure 1
References
Tarlov, A. R., J. E. Ware, S. Greenfield, E. C. Nelson, E. Perrin, and M. Zubkoff. 1989. The medical outcome study. Journal ofthe American Medical
Association 262: 925-930.
sqv1.3 An enhanced Stata logistic regression program
Joseph Hilbe, Editor, STB, FAX 602-860-1446
logiodd2 has been corrected to provide m-asymptotic influence statistics as described by Hosmer and Lemeshow (1989).
The new e option has been modified to provide only the basic goodness-of-fit statistics, Wald statistics, and partial correlations,
and the new i. option provides the influence and residual statistics. Unfortunately, in the current version, i. can only be specified
when there are 10 or fewer independent variables.
The important difference is that logiodd2 now adjusts for the number of covariate patterns of the independent variables.
For example, the data set
y xl x2 x3
1 10 1
1 110
0 011
0 10 1
1 110
consists of five observations but only three covariate patterns. The residual and influence statistics are a function of the number
of such patterns in the data set, the number of observations sharing the same covariate pattern, and the number of positive
responses within each pattern.