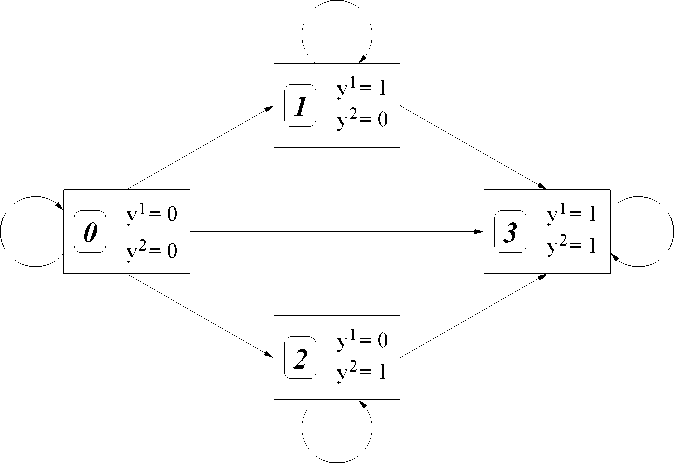
Figure 2: State-Transition Diagram for a Bivariate Survival Model
Conditions for simultaneous independence and Granger поп-causality can be
easily adapted from those introduced in Section 2, and take the particularly
simple form:
H1^2
h1→2
(Y
(.y
‰2 (Y1ΦY2
‰ =0
∕⅞ι =0
p = 0
Also the introduction of exogenous variables can be accounted for by simply
paralleling the solutions presented in Section 3. The same holds true as regards
the introduction of the lagged dependent variables yi,t-j', saturated and unsat-
urated models are defined as in Section 3, but a comment is in order: in this
case conditioning on the lagged endogenous variables is meaningful only when
the individual is in state 1 or 2, since when he is in state 0, conditioning on this
information induces no change in the transition probabilities, as state 0 cannot
be accessed from any other state of the model.
As for the asymptotic properties of the estimates and tests, notice that for
this model, even in the case of homogeneous populations, Proposition 5 does not
hold, since this model can be represented as a Markov chain with an absorbing
state corresponding to state 3. This means that N —> ∞ is needed in order
to ensure consistency and asymptotic normality of the estimates. Conversely,
T —> ∞ does not lead to the usual asymptotic properties. The intuition is that,
for any finite N, when T —> ∞ all individuals will eventually end up in the
16
More intriguing information
1. Økonomisk teorihistorie - Overflødig information eller brugbar ballast?2. What should educational research do, and how should it do it? A response to “Will a clinical approach make educational research more relevant to practice” by Jacquelien Bulterman-Bos
3. Learning-by-Exporting? Firm-Level Evidence for UK Manufacturing and Services Sectors
4. The name is absent
5. The Advantage of Cooperatives under Asymmetric Cost Information
6. Licensing Schemes in Endogenous Entry
7. Constrained School Choice
8. The name is absent
9. Segmentación en la era de la globalización: ¿Cómo encontrar un segmento nuevo de mercado?
10. The name is absent