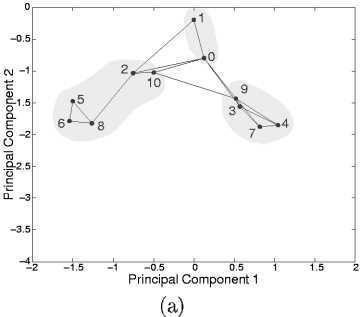
ω
10F ' ' ' ' ' :
9------ ---- ---- - ■
8- -- -
7------- ----- . _ —----
6- ■ --- ----- ∙ -
1 5- -
4- -
3------ ----- ------
2 - - -------- ∙ - —----- - - - -
1 . - - - - -
0 1000 2000 3000 4000 5000 6000
Time
(b)
Figure 8: The wall-following experiment: (a) a SOFM produced by the attention system at the end of a learning
episode, projected onto the first 2 principal components; the emergent clusters are highlighted; (b) the SOFM node
activation at the recall phase.
For example nodes 3,7, and 9 form a cluster for a wall
on one side and are also intermittently active in the
recall phase. Secondly, we see an emergent sequence
of activations as the robot moves around trying to
recall the information from the motor schemas: the
activations of nodes for wall perception on either side
(i.e. nodes 3-7-9, or nodes 2-6-8) are separated by ac-
tivations of nodes for no-wall perception (.i,e. nodes
0-1). This reflects our visual observations of the ac-
tual behaviour of the robot; for example, Figure 8(b)
corresponds to following a right wall, then no wall,
then following a right wall again, then no wall, then
following a left wall, etc.
Note that the activation dynamics here are differ-
ent than in the first experiment (Figure 3(b)), be-
cause of what is being modelled. In the first exper-
iment the behaviour modelled by the SOFM has a
true sense of sequence in it (moving the hand towards
a glass, picking it up, etc.); each node stores a part
of that sequence. In the wall-following experiment,
each node, or rather cluster of nodes, corresponds to
being in a particular perceptual ‘state’, and the mo-
tor skills are responsible for maintaining that state
(for example, fine-tuning to stay next to a wall on
the left). For this reason we do not have a situation
where one node wins consistently for a long period;
rather, the robot will keep (re)adjusting itself by ac-
tivating alternate nodes within the same cluster.
5.2 Results
As in the first experiment, we are also evaluating the
performance of the system on this task numerically;
we do this by calculating the ‘energy’ that the robot
acquires from the wall at particular orientations from
it. At the end of the recall episode we can look at
the accumulated energy as a measure of how well the
robot performs the wall-following task. Due to prac-
tical time limitations the amount of data available is
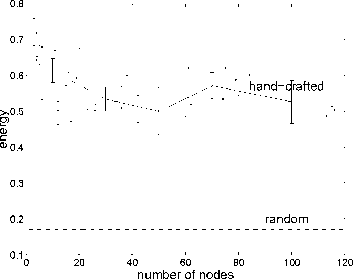
Figure 9: Evaluation of the recalled behaviour as a func-
tion of network size. Energy is measured from the sensors
at particular configurations from the wall, and compared
to energies acquired by a hand-crafted behaviour and a
random wandering behaviour. Raw data are also shown.
much smaller than in the first experiment, however
we believe it is sufficient for a reliable evaluation.
The dataset obtained in the learning phase was used
with different values of the novelty threshold to ob-
tain various SOFM networks for testing: around 15
different network sizes were produced, each repeated
between 2 and 5 times.
The energies acquired by the various networks are
shown in Figure 9, together with energies acquired
by a hand-crafted wall-following behaviour, and a
random wandering behaviour. We see that small
networks are preferred and that increasing them by
more than 30 nodes does not have a significant effect.
6. Discussion
We have presented an architecture that can represent
the sensory-motor experiences of a robot, in such
a way to enable recognition and reproduction of a
More intriguing information
1. Peer Reviewed, Open Access, Free2. Food Prices and Overweight Patterns in Italy
3. The name is absent
4. Should Local Public Employment Services be Merged with the Local Social Benefit Administrations?
5. The name is absent
6. The name is absent
7. Non Linear Contracting and Endogenous Buyer Power between Manufacturers and Retailers: Empirical Evidence on Food Retailing in France
8. A Location Game On Disjoint Circles
9. The Impact of Financial Openness on Economic Integration: Evidence from the Europe and the Cis
10. Analyse des verbraucherorientierten Qualitätsurteils mittels assoziativer Verfahren am Beispiel von Schweinefleisch und Kartoffeln