sub-pixel level. The projection of the local image models on this set of spatial patterns
is used as input data to the network, as described in the next paragraph.
Neural network training results
To estimate the HR pixels, it has been used a multi-layer perceptron (MLP) archi-
tecture [11], with two layers of neurons. The hidden layer is composed of 10 neurons
with hyperbolic tangent activation functions. These neurons are connected to a single
neuron in the output layer, with a linear activation function. The output of this neuron
provides a zero-mean, unit-variance estimation of the high-resolution central pixel.
Renormalization provides the final value. A diagram of the system is presented in fig-
ure 2 a).
The network has been trained using a conjugate gradient descent method on syn-
thetic data sets with various noise levels. The training data has been generated syn-
thetically from a set of 23 high-resolution images of urban scenes acquired by the
Quickbird satellite imaging system.
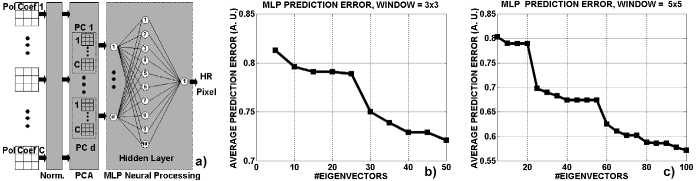
Fig. 2. a) Diagram of the superresolution neural processing stage; b) network average predic-
tion error, measured on a validation test set, as a function of the dimensionality of the network
input space for a 3x3 neighborhood; c) average prediction error for a 5x5 neighborhood.
The neural system has been trained for several input sizes, to study quantitatively
the effect on the predicting error of augmenting the input data with the value corre-
sponding to each sorted principal component. In figure 2 is presented the evolution of
this error as a function of the number of eigenvectors retained in the dimensionality
reduction step, for local windows of sizes 3x3 and 5x5. As it is apparent, the error de-
creases non-gradually, with almost flat zones interleaved with step decreases in the
prediction error. Furthermore, these step changes occurred also in zones where the
representational error of the dimensionality reduction step might be seen in principle
as negligible (see figure 1, for a 3x3 window), highlighting the inadequacy of an
MSE-based criteria in this case to select the dimension of the network input space.
Experimental Results
The accuracy and stability of the proposed method has been tested with both synthetic
and outdoor sequences of different image content. Here, we present the results of ap-
plying superresolution on synthetic image sequences with various noise levels, to en-
able the quantitative comparison, in terms of RMS, of the prediction error of different
More intriguing information
1. Heterogeneity of Investors and Asset Pricing in a Risk-Value World2. Ongoing Emergence: A Core Concept in Epigenetic Robotics
3. Fertility in Developing Countries
4. The name is absent
5. Mergers and the changing landscape of commercial banking (Part II)
6. The InnoRegio-program: a new way to promote regional innovation networks - empirical results of the complementary research -
7. The name is absent
8. The Values and Character Dispositions of 14-16 Year Olds in the Hodge Hill Constituency
9. Impact of Ethanol Production on U.S. and Regional Gasoline Prices and On the Profitability of U.S. Oil Refinery Industry
10. Modellgestützte Politikberatung im Naturschutz: Zur „optimalen“ Flächennutzung in der Agrarlandschaft des Biosphärenreservates „Mittlere Elbe“