methods. The sequences have been generated from a high-resolution image of an ur-
ban area acquired with the IKONOS satellite imaging system. This image has not
been used during the training process. From this image, two sequences of 25 images
(corresponding to 1s in the CCIR standard) were generated, one corresponding to the
noiseless case, and the second one corrupted highly by addition of Gaussian noise (σ
= 20 gray levels).
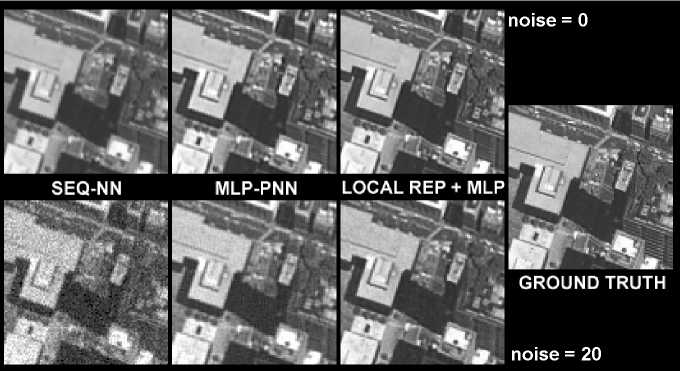
Fig. 3. Superresolution results, for a scaling factor of 2, on a synthetic sequence of an urban
area using different methods. In the first row, are presented the results for a noiseless input se-
quence. The second row contains the results for a sequence corrupted with Gaussian noise of σ
= 20 gray levels. Each column contains the results of a different method. The ground truth is
presented at the right of the image.
On figure 3 are presented the results obtained with three superresolution methods.
In the first column (SEQ-NN) are shown the results obtained estimating the HR pixels
as the value of the nearest projected sequence pixel. This method produces jagged
edges in the noiseless case, and no input data noise reduction. Both effects are re-
duced, as can be seen in the second column, when using the MLP-PNN technique,
with networks specialized in the appropriate noise range. Finally, the use of the pro-
posed method, with 3x3 neighborhoods and 40 eigenvectors, improves both image
definition and noise rejection at all input noise levels, as can be seen in the third col-
umn of the figure. These perceptual results are supported by the RMS error figures
reported in table 2.
Table 2. High-resolution RMS reconstruction errors for several methods
ZOOM |
SEQ-NN |
MLP-PNN |
LOCALREP+MLP | |
noiseless sequence (σ=0) |
19.39 |
13.04 |
________11.24 |
________________8.28 |
noisy sequence (σ=20)_____ |
24.08 |
23.58 |
________14.60 |
_______________11.06 |
More intriguing information
1. The name is absent2. Computational Experiments with the Fuzzy Love and Romance
3. Prizes and Patents: Using Market Signals to Provide Incentives for Innovations
4. Kharaj and land proprietary right in the sixteenth century: An example of law and economics
5. The name is absent
6. Evolution of cognitive function via redeployment of brain areas
7. The name is absent
8. If our brains were simple, we would be too simple to understand them.
9. 5th and 8th grade pupils’ and teachers’ perceptions of the relationships between teaching methods, classroom ethos, and positive affective attitudes towards learning mathematics in Japan
10. Strengthening civil society from the outside? Donor driven consultation and participation processes in Poverty Reduction Strategies (PRSP): the Bolivian case