events, occurring every 300 generations, we find brief spikes in
activity for each of the multi-objective problems. This
demonstrates that changes in who a species interacts with in a
multi-objective environment can influence the adaptive options
taken (i.e. the evolutionary trajectory) by that species. In
particular, these interaction changes are able to induce new
positive adaptations that would not have taken place otherwise.
To assess the significance of this effect, we use a non-
parametric statistical test (Mann-Whitney U Test) and calculate
the confidence that a distribution of ΔPh outputs (at time t) has
a median value that is greater than the distribution at the time t-
1. For all of the MOPs (but none of the SOPs) we find that at
least one of the migration events has a significant increase in
median activity (p<0.01). On the other hand, there is never a
statistically significant increase in activity at other (non-
migration) positions in the time series.
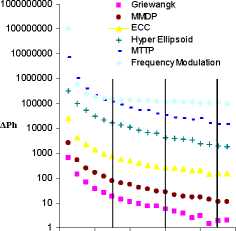
0 200 400 600 800 1000
t
Figure 4: Time series of evolutionary activity for ESIM
evolving on a set of single objective (left) and multi-objective
(right) problems with large migration events (swaps=100) at
every 300 generations.
1000000000
100000000
10000000
1000000
100000
APh 10000
1000
100
10
1
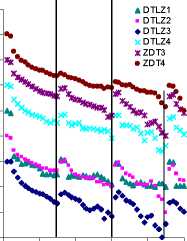
0 200 400 600 800 1000
t
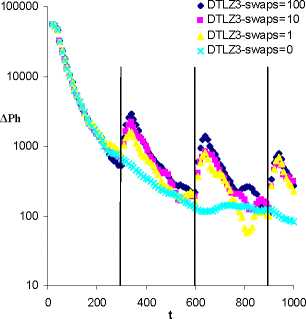
Figure 6 Time series of evolutionary activity for ESIM
evolving on DTLZ3 with different migration sizes (swaps=0, 1,
10, 100) which occur at every 300 generations. Results are
averaged over 50 runs.
The results in Figure 6 look at the influence of the migration
size on evolutionary activity. Results are only shown for
DTLZ3 however similar behavior occurs for the other MOPs in
Table 1. This figure confirms that when migration events are
prevented between islands in MOO, the species converge
without the periodic spikes in evolutionary activity. This
figure also demonstrates that the migration size has very little
impact and that even the smallest migrations can cause periods
of increased evolutionary activity. From this, we speculate
that migration events are initiating a cascade of activity such
that large portions of the population can be driven to
reorganize into new meta-stable states even when the initial
size of the migration event is small.
4 Discussion
4.1 Coevolution in multi-objective optimization
Coevolution describes a phenomena where species influence
the fitness landscapes and consequently the evolutionary
trajectory of other species. The more general phenomena of
coupled dynamics, in which coevolution is a special case,
occurs in many contexts and is commonplace in driven
systems of interacting components. For instance, an EA
population is typically able to converge to a single attractor (or
attractor basin) within a single objective fitness landscape as a
consequence of selective and recombinative operators. On the
other hand, coevolution involves a different form of coupled
dynamics where individual species are not driven to the same
attractors but instead mutually influence each other’s uniquely
defined fitness landscapes, which in turn can drive the
evolution of individual species in unique directions. Multi-
objective problems provide an environment for optimization
where individuals are also driven to occupy unique phenotypes
(e.g. along the Pareto Front). We suspect that this aspect of
multi-objective problems could be directly responsible for the
intransitivity of Pareto dominance as well as the
coevolutionary behaviors reported here.
Coevolution through Constraints: ESIM creates a
coevolutionary process that operates by constraining the future
adaptive options within a fitness landscape. In particular, the
ranking interactions used to define fitness in ESIM act by
constraining which movements in objective space are observed
as fitness improvements, which movements are neutral and
which are negative. This is dissimilar from conventional
models of coevolution that are based on explicit acts of
cooperation or competition. In future studies, we intend to
explore whether “constraints-based” coevolutionary models
provide unique opportunities for understanding coevolution.
Coevolution with single objectives: Although our results did
not find coevolutionary dynamics in SOO, this does not mean
that such a phenomenon is not possible. In [2] it is shown
how fitness ranking calculations that are restricted to occur
through sparsely connected interaction networks can be used
to generate a contextual definition of fitness that allows two
connected individuals to flip their ranking order when changes
More intriguing information
1. The name is absent2. Flatliners: Ideology and Rational Learning in the Diffusion of the Flat Tax
3. The name is absent
4. What Lessons for Economic Development Can We Draw from the Champagne Fairs?
5. Optimal Private and Public Harvesting under Spatial and Temporal Interdependence
6. Voluntary Teaming and Effort
7. The purpose of this paper is to report on the 2008 inaugural Equal Opportunities Conference held at the University of East Anglia, Norwich
8. Dynamiques des Entreprises Agroalimentaires (EAA) du Languedoc-Roussillon : évolutions 1998-2003. Programme de recherche PSDR 2001-2006 financé par l'Inra et la Région Languedoc-Roussillon
9. Studies on association of arbuscular mycorrhizal fungi with gluconacetobacter diazotrophicus and its effect on improvement of sorghum bicolor (L.)
10. Putting Globalization and Concentration in the Agri-food Sector into Context