102
with the same prognosis.
Appendix
Gibbs Sampling Scheme
Any set of imputed parameters θι,..., θn corresponds to a partition p = (Si,..., S⅛)
of the indices {1,..., n}. Let θ*,..., θ*κ the unique values in the sample in order of
appearance and define Sk = {i '■ θi = θ*k}.
Consider the Dirichlet process mixture model (Antoniak, 1974) in (3.9). Let
д~г — (flɪ,...,0i-ι,θi+1, ...,θn) denote all values but θi. Let K~l be the number of
unique values in θ~∖ let θ↑~∖ ..., θ*1^i be these different values in order of appearance
and p~l be the partition implied by θ~l. Use equation (4.5) with the n—1 observations
and let the observation i be the future observation (exchangeability allows us to
permutate the indices) to get:
κ~i
p[θi I θ~i] = fκ-41(p-i)g0(θi) + ∑ Λ(p-‰-O,
fc=l
where δx is a pointmass at x and go is the pdf corresponding to Go- Therefore,
p[θi I θ~i,yn] <xp[yn I θi,θ~i]p[θi I θ~i]
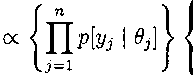
κ~i `
⅛.+ι(∏G0(¾) + ,
fc=l
κ~i
<xp[Vi I θl]fκ→+1{p~l')Go{θi') + V fk(p~l)p[yi I θi]δθ*-i(θi)
• ∙ K
fc=l
Using (4.5) we get:
κ~i / +1 V
p[θi I θ~∖ yn] oc ⅛p[yi I 0i]Go(0l) + £ #Sfc ) p[yi ∣ Λ-i(θi),
where I is the value of the categorical covariate (in our particular, the prognosis)
corresponding to the ith experimental unit (patient).
More intriguing information
1. The name is absent2. The name is absent
3. The name is absent
4. The demand for urban transport: An application of discrete choice model for Cadiz
5. Transfer from primary school to secondary school
6. The name is absent
7. On Dictatorship, Economic Development and Stability
8. The name is absent
9. The name is absent
10. The name is absent