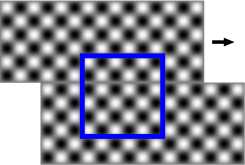
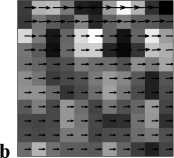
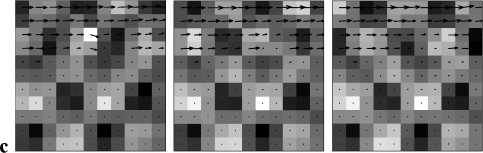
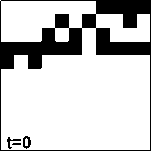
Fig. 5. Piece-wise smooth optical flow estimation - motion
segmentation. (a) The plaid pattern stimulus providing a lin-
ear motion boundary. (b) Optical flow estimate with discon-
tinuity units being disabled. Scanned output sequence of (c)
the optical flow and (d) the discontinuity network.
In the second example, a stimulus with a less complex
motion boundary was applied (Figure 5a). The stimulus
consisted of two tightly joined, identical sinewave plaid pat-
terns. One pattern was stationary while the other one moved
horizontally to the right, thus forming a linear motion dis-
continuity. In a first instance, the motion discontinuity units
were disabled. As shown in Figure 5b, the smoothness con-
straint forces the flow field to be smooth, thus assigning
uniform motion to the moving plaid (as desired) but also
to the stationary pattern. Enabling the discontinuity units
(Figure 5c,d), however, leads to a clear separation of the
two motion sources and thus to the segmentation of the vi-
sual scene in two areas of distinct, piece-wise smooth op-
tical flow. Note, that this is hardly possible without using
the error signal generated by the brightness constraint vio-
lations.
5. DISCUSSION
The presented focal-plane implementation is an example of
the successful physical translation of collective computa-
tional principles in recurrent network architectures. The im-
plementation provides near-optimal solutions to the compu-
tationally hard problem of motion segmentation. The chip’s
dynamical reassessment of its connectivity pattern did prove
to enhance optical flow estimation as compared to previ-
ous implementations with no [2], or constant interaction
strengths [3, 1].
An increased array size of the processor will certainly
improve the apparent quality of its motion estimates as well
as its ability to detect motion boundaries. Furthermore, lo-
cal interactions of the motion discontinuity units in a soft-
WTA (winner-takes-all) manner can adapt the effective thresh-
old locally to reduce the present susceptibility to a fixed
threshold value. Nevertheless, the current implementation
is already close to the feasible limits of focal-plane imple-
mentation. Any increase in connectivity might require to
split up the system into multiple chips.
6. REFERENCES
[1] A. A. Stocker and R. J. Douglas, “Computation of
smooth optical flow in a feedback connected analog net-
work,” in Advances in Neural Information Processing
Systems 11, M. S. Kearns, S. A. Solla, and D. A. Cohn,
Eds., Cambridge, MA, 1999, pp. 706-712, MIT Press.
[2] R. Etienne-Cummings and P. Mueller, “A focal plane
visual motion measurement sensor,” Trans. on Circuits
and Systems I, vol. 44, no. 1, pp. 55-66, January 1997.
[3] J. Tanner and C.A. Mead, “An integrated analog opti-
cal motion sensor,” in VLSI Signal Processing, 2, S.-Y.
Kung, R. Owen, and G. Nash, Eds., p. 59 ff. IEEE Press,
1986.
[4] J. Hutchinson, C. Koch, J. Luo, and C. Mead, “Comput-
ing motion using analog and binary resistive networks,”
Computer, vol. 21, pp. 52-64, March 1988.
[5] J. Kramer, R. Sarpeshkar, and C. Koch, “Analog
VLSI motion discontinuity detectors for image segmen-
tation,” in Intl. Symposium on Circuits and Systems.
IEEE, 1996, pp. 620-623.
[6] J.J. Hopfield and D.W. Tank, “Neural computation of
decisions in optimization problems,” Biological Cyber-
netics, , no. 52, pp. 141-152, 1985.
[7] A. A. Stocker, Constraint Optimization Networks for
Visual Motion Perception - Analysis and Synthesis,
Ph.d. thesis no. 14360, Swiss Federal Institute of Tech-
nology ETHZ, Ziirich, Switzerland, September 2001.
[8] S. Geman and D. Geman, “Stochastic relaxation, Gibbs
distributions, and the Bayesian restoration of images,”
IEEE Trans. on Pattern Analysis and Machine Intelli-
gence, vol. 6, no. 6, pp. 721-741, November 1984.
[9] T. Delbruck, “Bump circuits,” Tech. Rep. CNS Memo
26, Caltech, Pasadena, California 91125, May 1993.
II - 335
More intriguing information
1. Towards a framework for critical citizenship education2. The name is absent
3. Expectations, money, and the forecasting of inflation
4. Measuring Semantic Similarity by Latent Relational Analysis
5. TLRP: academic challenges for moral purposes
6. How Low Business Tax Rates Attract Multinational Headquarters: Municipality-Level Evidence from Germany
7. Behaviour-based Knowledge Systems: An Epigenetic Path from Behaviour to Knowledge
8. Why Managers Hold Shares of Their Firms: An Empirical Analysis
9. The name is absent
10. PEER-REVIEWED FINAL EDITED VERSION OF ARTICLE PRIOR TO PUBLICATION