object. If a salient object is observed in the robot’s
view, the robot shifts its gaze direction from the care-
giver’s face to the object based on the visual atten-
tion mechanism. When visual attention succeeds,
the robot evaluates it and then learns the sensori-
motor coordination between the inputs I and θ, and
the output ∆θ based on the mechanism of learning
with self-evaluation.
Since visual attention does not always correspond
to joint attention, the robot may have two kinds of
learning situations: correct learning situations for
joint attention and incorrect ones.
• In the former case, that is when the robot attends
to the same ob ject that the caregiver attends to,
the robot can acquire the appropriate sensorimo-
tor coordination for joint attention.
• In the latter case, that is when the robot attends
to the different ob ject from that the caregiver at-
tends to, the robot cannot find the sensorimotor
correlation since it is supposed that the object
position that the robot attends to changes ran-
domly.
Therefore, the incorrect learning data would be ex-
pected to be statistically lost as outliers through the
learning, and the appropriate sensorimotor correla-
tion for joint attention survives in the learning mod-
ule. Furthermore, by activating the learning module,
which has already acquired the sensorimotor coordi-
nation, to attend to an object instead of the visual
attention mechanism, the robot can reduce the pro-
portion of the incorrect data and acquire more appro-
priate coordination for joint attention. Through the
above learning process, the robot acquires the ability
of joint attention without a controlled environment
nor external task evaluation.
2.2 A constructive model for joint attention
The proposed constructive model for joint attention
is shown in Figure 3. As described above, the robot
receives the camera image I and the angle of the
camera head θ as the inputs and outputs the motor
command to the camera head ∆θ. The following
modules corresponding to (a) visual attention and
(b) learning with self-evaluation constitute the pro-
posed model.
(a-1) Salient feature detector extracts distinguishing
image areas from I .
(a-2) Visual feedback controller receives the detected
image features and outputs V F ∆θ to attend to
an interesting object.
(b-1) Internal evaluator drives the learning mechanism
in the learning module when the robot attends to
the interesting object.
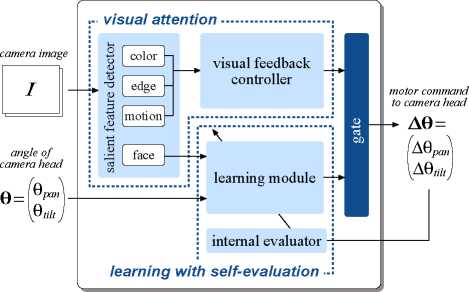
Figure 3: A constructive model for joint attention based
on visual attention and self learning
(b-2) Learning module receives the image of the care-
giver’s face and θ as the inputs and outputs
LM∆θ. This module learns the sensorimotor co-
ordination when the internal evaluator triggers
it.
In addition to these modules, the proposed model
has another one to arbitrate the output of the robot.
(c) Gate makes a choice between V F ∆θ and LM ∆θ,
and outputs ∆θ as the robot’s motor command.
The following sections explain these modules in de-
tail.
2.2.1 Salient feature detector
The salient feature detector extracts distinguishing
image areas in I by color, edge, motion, and face
detectors. The color, edge, and motion detectors ex-
tract the objects (i = 1, . . . , n) which have bright col-
ors, complicated textures, and motions, respectively.
Then, the salient feature detector selects the most
interesting object itrg among the extracted objects
by comparing the sum of the interests of all features.
itrg = argmax(α1ficol + α2fiedg + α3fimot), (1)
i
where ficol , fiedg , and fimot indicate the size of the
bright color area, the complexity of the texture, and
the amount of the motion, respectively. The coeffi-
cients (α1, α2, α3) denote the degrees of the interests
of three features, that are determined according to
the robot’s characteristics and the context. At the
same time, the face detector extracts the face-like
stimuli of the caregiver. The detection of face-like
stimuli is a fundamental ability for a social agent;
therefore, it should be treated in the same manner as
the detection of the primitive features. The detected
primitive feature of the ob ject itrg and the face-like
one of the caregiver are sent to the visual feedback
controller and the learning module, respectively.
More intriguing information
1. Mergers under endogenous minimum quality standard: a note2. PRIORITIES IN THE CHANGING WORLD OF AGRICULTURE
3. The name is absent
4. Improvement of Access to Data Sets from the Official Statistics
5. THE EFFECT OF MARKETING COOPERATIVES ON COST-REDUCING PROCESS INNOVATION ACTIVITY
6. Inhimillinen pääoma ja palkat Suomessa: Paluu perusmalliin
7. Personal Income Tax Elasticity in Turkey: 1975-2005
8. The name is absent
9. Non-farm businesses local economic integration level: the case of six Portuguese small and medium-sized Markettowns• - a sector approach
10. Internationalization of Universities as Internationalization of Bildung