stage I
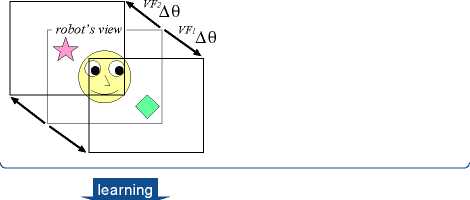
stage II
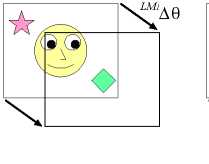
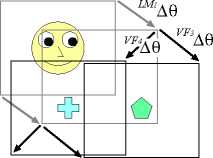
learning
stage III
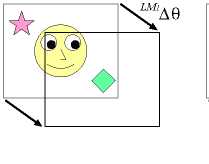
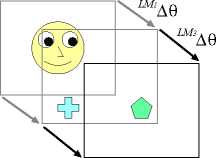
Figure 4: The incremental learning process of joint atten-
tion. The robot acquires the sensorimotor coordination
of lm 1 ∆θ and lm3 ∆θ.
At the middle right of Figure 4, if the object that
the caregiver attends to is out of the robot’s first
view, the robot can find the object not at the cen-
ter but at the periphery of its view by LM1 ∆θ.
Then, the robot outputs VF3∆θ or VF4∆θ to at-
tend to an interesting object in the field of its
view case by case. When visual attention suc-
ceeds, the robot learns the sensorimotor coordi-
nation in each case.
stage III: In the final stage, the robot has acquired
the complete ability of joint attention owing to
the learning in stages I and II. At the bottom of
Figure 4, the robot can identify the object that
the caregiver attends to by producing LM1 ∆θ
and LM3 ∆θ even if the object is observed in the
field of the robot’s first view or not. The sensori-
motor coordinations of LM1 ∆θ and LM3 ∆θ have
been acquired through the learning in stages I
and II because each of VF1∆θ and VF3∆θ had
the sensorimotor correlation for joint attention
The above incremental learning process of the
robot’s joint attention can be regarded as equiva-
lent to the staged developmental process of an in-
fant’s one shown in Figure 1. The stages I, II, and
III of the robot correspond to the infant at the 6th,
12th, and 18th month, respectively. In addition, it is
supposed in the cognitive science that the embedded
mechanisms of the robot, visual attention and learn-
ing with self-evaluation, are also prepared in the in-
fant inherently (Bremner, 1994). Therefore, the sim-
ilarity of the developmental phenomena and the em-
bedded mechanisms between the robot’s joint atten-
tion and the infant’s one suggests that the proposed
constructive model could explain the developmental
mechanism of the infant’s joint attention.
3. Experiment
3.1 Experimental setup
It is examined whether an actual robot can acquire
the ability of joint attention based on the proposed
model in uncontrolled environments including mul-
tiple salient objects. An experimental environment
is shown in Figure 5 (a), and the left camera image
of the robot is shown in (b). Some salient objects
are places in the environment at random positions.
The caregiver who sits in front of the robot attends
to one object (in Figure 5, it attends to the object in
its hand). The robot has two cameras and can turn
them to pan and tilt simultaneously. The robot re-
ceives the camera image and detects the caregiver’s
face and the objects by the salient feature detector as
shown in Figure 5 (b). In this experiment, the robot
has the degrees of the interests of the image features
(α1, α2, α3) = (1, 0, 0) in Eq. (1). The thresh-
old of the success of visual attention is defined as
dth = (the width of the camera image)/6 in Eq. (3).
To execute the simulated learning, we acquired 125
data sets, each of which included
• the left camera image (in which the caregiver’s
face was extracted as a window of which size is
30 × 25 pixels) and the angles of the camera head
(pan and tilt) when the robot attended to the
caregiver’s face, and
• the motor command for the camera head to shift
its gaze direction from the caregiver to the object
that the caregiver attended to
in advance. Then, in each trial, we took one data
set from the above and placed other salient objects
at random positions in the simulated environment.
The number of input, hidden, and output units of
the learning module were set 752 (30 × 25 + 2), 7,
and 2, respectively. Under this condition, the robot
repeated alternately the trial and the learning based
on the proposed model.
More intriguing information
1. Rural-Urban Economic Disparities among China’s Elderly2. Heavy Hero or Digital Dummy: multimodal player-avatar relations in FINAL FANTASY 7
3. Thresholds for Employment and Unemployment - a Spatial Analysis of German Regional Labour Markets 1992-2000
4. Standards behaviours face to innovation of the entrepreneurships of Beira Interior
5. APPLYING BIOSOLIDS: ISSUES FOR VIRGINIA AGRICULTURE
6. The name is absent
7. Distortions in a multi-level co-financing system: the case of the agri-environmental programme of Saxony-Anhalt
8. LOCAL PROGRAMS AND ACTIVITIES TO HELP FARM PEOPLE ADJUST
9. The name is absent
10. Inflation Targeting and Nonlinear Policy Rules: The Case of Asymmetric Preferences (new title: The Fed's monetary policy rule and U.S. inflation: The case of asymmetric preferences)