-5
Unemployment Rate
0.15
0.1
0.05
0
-0.05
-0.1
-0.15
-0.2
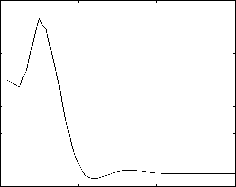
0246
Short-term Unemployment
0
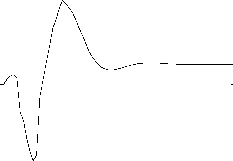
0246
Years
λ
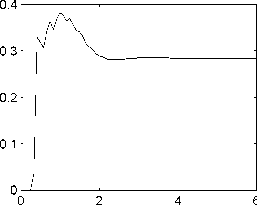
Long-term Unemployment
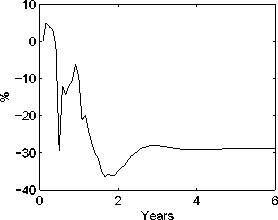
Fig. 3. Model Based Predictive Control. (% deviation from baseline, except λ).
3.3 Model Based Predictive Control
Model based predictive control (MBPC) is a suite of control methods where a
linear model is used to predict the future output of the full nonlinear model;
and the control rule is developed from the prediction is applied to the full non-
linear model. As with the linear-quadratic approach, the control is developed
dynamically (at each time-step) and is applied to the full nonlinear model at
the same time-step; but unlike the linear-quadratic approach the linear model
is regenerated dynamically as part of the control rule generation. Details of the
methods can be found in Rossiter (2003) and we use the approach developed
in Herbert and Bell (2004).
The MBPC approach attempts to overcome the accuracy of the linear model
in the linear-quadratic method by using a time-varying linear model. In the
results here, we also add a learning component so the linear model adjusts
according to its difference in predicted output from the nonlinear model (Her-
bert, 1998).
The MBPC approach also allows for explicit constraints to be added in the
control rule generation. The constraints can be placed on the level and rate of
change of the output as well as the control. Such constraints are much more
realistic with labor market models where there are plausible policy strategies
and un-modelled components.
MBPC is more complex mathematically than the linear-quadratic approach.
More intriguing information
1. Initial Public Offerings and Venture Capital in Germany2. The effect of globalisation on industrial districts in Italy: evidence from the footwear sector
3. The name is absent
4. The name is absent
5. Barriers and Limitations in the Development of Industrial Innovation in the Region
6. Job quality and labour market performance
7. Constructing the Phylomemetic Tree Case of Study: Indonesian Tradition-Inspired Buildings
8. The name is absent
9. Qualifying Recital: Lisa Carol Hardaway, flute
10. An institutional analysis of sasi laut in Maluku, Indonesia