3-2 where, as expected, a concentration process near the most important urban centres is
taking place.
In conclusion, the adoption of the SOM as a tool to investigate the different dynamics
seems fruitful and opens new research directions. In fact the different Codebooks may
be interpreted in a “if then else “ approach: given these surrounding conditions at time t
at time t+1 the dynamics will change in this way.
6. The prediction through Supervised ANN
The NN which generates prediction on land use dynamics is Supervised (SANN).
Trained on the base of Input/Output examples the NN is able to reproduce the expected
Output starting from the same Input. This means that the NN should learn, from the set
of cells which change their land use in the time lag considered, the connections between
the final state at time t+1 (the target) and the local and neighbouring conditions at
time t.
The record supplied to the SANN contains 6 input variables and 3 output ones. The
input describe the state of the cell and of its neighbourhood at the time t, the output
variables represent only the cell state at the time t+1.
For the implementation of supervised NN, it is crucial to select “good examples ”to feed
the network. Therefore the records have been split into two different sets (Fig. 4).
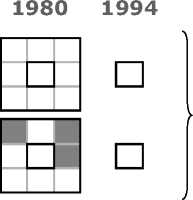
excluded
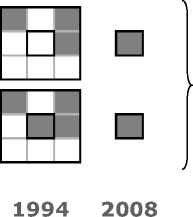
ANN, training and prediction
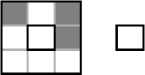
ANN, prediction
Figure 4 - The record sets used for the different processing phases
10
More intriguing information
1. Consumption Behaviour in Zambia: The Link to Poverty Alleviation?2. The Clustering of Financial Services in London*
3. Human Rights Violations by the Executive: Complicity of the Judiciary in Cameroon?
4. Voting by Committees under Constraints
5. CONSUMER ACCEPTANCE OF GENETICALLY MODIFIED FOODS
6. The Importance of Global Shocks for National Policymakers: Rising Challenges for Central Banks
7. Subduing High Inflation in Romania. How to Better Monetary and Exchange Rate Mechanisms?
8. Ability grouping in the secondary school: attitudes of teachers of practically based subjects
9. The name is absent
10. Nonparametric cointegration analysis