ment get negative reinforcement and vice-versa, or an extra-
dimensional shift, in which the dimension is changed on
which the reinforcement is given, in the example from white
to large. It turns out that adults and older children are faster
at learning the reversal-shift condition, while young children
and animals are faster at the extra-dimensional shift. Figure
12 shows the results of an experiment by Kendler and Ken-
dler (1959). The ACT-R model of adult behavior uses the
тЗ
<υ
тз
<υ
CS
H
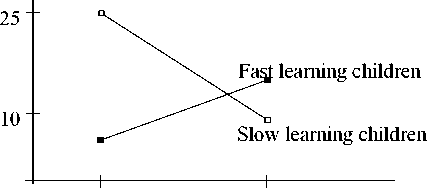
reversal extra-dimensional
Figure 12: Trials needed to learn the discrimination-shift
same 8 production rules as used in the beam-task, imple-
menting the property-retrieval and find-fact-on-feedback
strategies. The small-child/animal model uses only 2 of the 8
production rules, implementing a limited find-fact-on-feed-
back strategy. The results of these models are shown in fig-
ure 13. Although the models do not mimic the subjects
results precisely, the general effects are in the same direc-
tion.
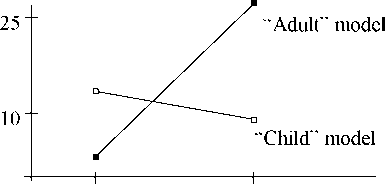
reversal extra-dimensional
Figure 13: Results of the ACT-R model on the discrimina-
tion-shift task
Despite the fact that the discrimination-shift task is gener-
ally not considered to be an insight problem, it nevertheless
requires the subject to notice that something has changed,
and to discover the new relations. So it can be seen, in a
sense, as an elementary insight problem.
Discussion
The ACT-R model addresses some of the questions posed by
the dynamic growth model. ACT-R itself already answers
some of the questions: how knowledge is represented, and
what the effects of success or failure are. The question how
new knowledge can be acquired isn’t fully answered by the
ACT-R theory. The model presented here is an attempt to
supply the first steps to an answer, since the strategies imple-
mented in the model can learn several different tasks. The
discrimination-shift model is interesting in the sense that it
shows that an adult model can be changed into a child model
only by deleting some productions rules. This shows that the
general rules themselves aren’t hard-wired, but must be
learned as well, perhaps using the same mechanisms as
needed for task-specific knowledge. So a next step will be to
find out what other general rules people employ, and how
general rules themselves can be learned.
Conclusions
So, what should we do when we have to solve a new prob-
lem? Just search for a solution or just think hard and hope for
an insight? According the dynamic growth model, the most
rational thing to do is a proper alternation of the two. The
model allows manipulation of several parameters, like the
value of the goal as has been discussed. But other parameters
can be changed as well, for instance the amount of prior
knowledge, importance of level 2 knowledge, etc., allowing
for new predictions.
The ACT-R model shows how aspects of the dynamic
growth model can be implemented using real knowledge rep-
resentations instead of variables. Although both knowledge
needed for reflection and for search are represented by pro-
duction rules, there are differences between the two: reflec-
tion knowledge is general, and is relatively costly to use, and
search knowledge is task-specific and cheap, but possibly
insufficient to reach the goal.
References
Anderson, J.R. (1990). The adaptive character of thought.
Hillsdale, NJ: Lawrence Erlbaum.
Anderson, J.R. (1993). Rules of the Mind. Hillsdale, NJ:
Lawrence Erlbaum.
Davidson, J.E. (1995). The suddenness of insight. In R.J.
Sternberg & J.E. Davidson (Eds.), The nature of insight.
Cambridge, MA: MIT Press.
Kendler, T.S. & Kendler, H.H. (1959). Reversal and nonrever-
sal shifts in kindergarten children. Journal of experimental
psychology, 58, 56-50.
Lebiere, C. (1996). ACR-R 3.0: A users manual. Pittsburgh,
MA: Carnegie Mellon University.
Mayer, R.E. (1995). The search for insight. In R.J. Sternberg
& J.E. Davidson (Eds.), The nature of insight. Cambridge,
MA: MIT Press.
Newell, A. & Simon, H.A. (1972). Human problem solving.
Englewood Cliffs, NJ: Prentice-Hall.
Norman, D.A. (1993). Things that make us smart. Reading,
MA: Addison-Wesley.
Ohlsson, S. (1984). Restructuring revisited II. An information
processing theory of restructuring and insight. Scandina-
vian Journal of Psychology, 25, 117-129.
Taatgen N.A. (1996). Learning and revising task-specific
rules in ACT-R. In U. Schmid, J. Krems & F. Wysotzki
(Eds.), Proceedings of the First European Workshop on
Cognitive Modeling (report no. 96-39, pp. 31-38). Berlin,
Technische Universitaet Berlin, Fachbereich Informatik.
Van Geert, P. (1994). Dynamic systems of development:
Change between complexity and chaos. London: Harvester
Wheatsheaf.
More intriguing information
1. Factores de alteração da composição da Despesa Pública: o caso norte-americano2. Innovation and business performance - a provisional multi-regional analysis
3. THE CHANGING RELATIONSHIP BETWEEN FEDERAL, STATE AND LOCAL GOVERNMENTS
4. The name is absent
5. The name is absent
6. The name is absent
7. Om Økonomi, matematik og videnskabelighed - et bud på provokation
8. IMPACTS OF EPA DAIRY WASTE REGULATIONS ON FARM PROFITABILITY
9. Mean Variance Optimization of Non-Linear Systems and Worst-case Analysis
10. Evidence of coevolution in multi-objective evolutionary algorithms