O decreases. For action 1 and action 2, at the be-
ginning, the primed sensation is set as a long vector
in which every element- is zero. After taking an ac-
tion, the current sensation is very different from the
initial sensation. That is, the novelty value is high.
We can see from Fig. 3 that at first- several steps,
the Q-Values of these two actions increase. However,
after we update the primed sensation, the primed
sensation will be the same as the actual sensation
if the robot- takes the action again. Then the nov-
elty becomes zero and Q-valuc decreases. After a
long time training (300 steps), the robot- can predict
the actual sensation of next- step whatever action it
takes. So the Q-valuc of each action converges to
the same value (0). This means each action has the
same probability to be chosen. The right- part- of
Fig. 3 shows the number of each action in different-
time frames (60 steps in each time frame). At- the
beginning, action 0 has a larger Q-valuc, according
to Boltzmann exploration, it- has more chance to be
chosen. The probability of action 1 and action 2 is
almost- the same. After 300 steps, the Q-valuc of each
action is nearly equal, so the numbers of each action
are close. The experiment- shows that because of ha-
bituation effect-, the robot- loses the interest- of any
action after exploration and just- chooses an action
randomly.
1
0
-1
1
0
-1
1
0

-1∣-----------------------------------------------
1 5 10 15 20 25
Action 0 (Stare)
5 10 15 20 25
I---- Action 1 (Turn Left) I
5 10 15 20 25
Action 2 (Turn Right)
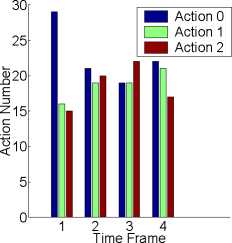
Action number
Time
Q-value vs time
Figure 3: Habituation effect. In the left- part: the 1st,
2nd and 3rd plots correspond to the Q-value of action 0,
action 1, and action 2 respectively. In the right- part, the
frequency of actions in different- time frames.
4-2 Integration of novelty and immediate
reward
After the above experiment-, we began to issue re-
wards. For example, when the robot- turns left-, hu-
man teachers give it- a positive reward (1). For other
two actions, negative rewards (-1) will be issued.
Then the actual reward the robot- receives is an inte-
gration of novelty and immediate reward. For action
0 and action 2, the Q-values change in the same way
as in experiment- 1, converging to 0. The Q-valuc of
action 1 is always positive because we keep issuing
positive rewards. As we can see in the left- part- of
Fig. 4, at the beginning, the Q-valuc of action 0 is
the largest- and the robot- takes the action with a high
probability. After training, the Q-valuc of action 1 is
much larger than that of other actions. As shown in
Fig. 4, gradually, action 1 is chosen the most- often.
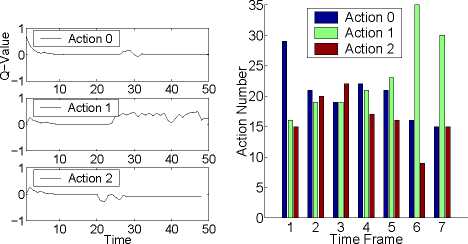
Q-value vs time
Action number
Figure 4: Integration of novelty and immediate reward.
4-3 Increase novelty with a moving object

Figure 5: Simulation of a moving object.
In order to show novelty preference, a moving toy
is added to the simulation environment- after exper-
iment- in Fig. 3. The testing image is shown in Fig.
5. Every time when the robot- is in the state with
the absolute viewing angle 0, one of these images is
generated randomly. Thus, the primed sensation of
action 0 is always different- from the actual sensation.
As shown in left- part- of Fig. 6, the Q-valuc of action
0 is positive because of high novelty. In contrast-, the
Q-Values of action 1 and action 2 are more near to
zero. After training, the robot- found that staying
with viewing angel of east- is the most- interesting.
So the action 0 is chosen the most- often.
4-4 Suppress novelty with immediate re-
wards
After the third experiment- , we issued positive re-
wards to action 2 (turn right), and negative rewards
to action 0. Thus, even though the novelty is high
when the robot- stares at a moving object-, the imme-
diate rewards suppress the novelty. Gradually, the
Q value of action 2 increases. As shown in Fig. 7,
after training, the robot- almost- chooses only action
2.