0.08
0.075
0.07
0.065
0.06
0.055
0.05
0.045
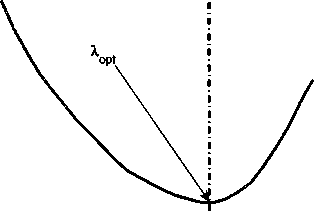
0.04-------------,-------------'-------------1-------?-----,-------------1~
0 5 10 15 20 25
Regularization coefficient λ
30
Figure 4.5: The variation estimation error for various regularization factors λ.
4.4 Determining the regularization coefficient λ
Consider ^-regularization problem,
min ∣∣τ∣∣ι + λ∣∣Aa? — fe∣∣2∙ (4-13)
When λ is very small, λ∣∣Ax — b∖∣2 would be small compared to the ^ɪ-norm term,
∣∣x∣∣ι and does not affect objective function dramatically. Thus, norm-one term
H:r∣∣ι is the main component that determines the solution of the regularization
problem; the solution tends to be sparse. In the other hand, when λ is very large,
λ∣∣√Lr — fe∣∣2 would be large compared to the norm-one term, ∣∣x∣∣ι, and small
changes in ∣∣√4τ — b∣∣2 result in large changes in objective function. In general, λ
balances between sparsity (Д-norm term) and fitting to measurements (∕⅛-norm
term).
Measurement noise and sparsity of the vector x are two major components
51