W. K. Hardle, R. A. Moro, and D. Schafer
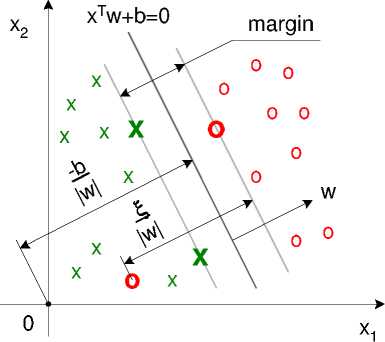
Fig. 4. The separating hyperplane xτw + b = 0 and the margin in a non-separable
case. The observations marked with bold crosses and zeros are support vectors. The
hyperplanes bounding the margin zone equidistant from the separating hyperplane
are represented as xτw + b = 1 and xτw + b = -1.
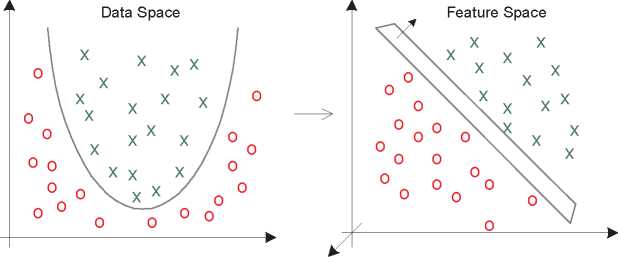
Fig. 5. Mapping from a two-dimensional data space into a three-dimensional space
of features R2 → R3 using a quadratic kernel function K(xi, xj) = (xiτxj)2. The
three features correspond to the three components of a quadratic form: x1 = x21, x2 =
√2x1x2 and X3 = x2, thus, the transformation is Ψ(x1, x2) = (x2, vz2x1x2, x2)τ. The
data separable in the data space with a quadratic function will be separable in the
feature space with a linear function. A non-linear SVM in the data space is equivalent
to a linear SVM in the feature space. The number of features will grow fast with d
and the degree of the polynomial kernel p, which equals 2 in our example, making
the closed-form representation of Ψ such as here practically impossible
More intriguing information
1. Infrastructure Investment in Network Industries: The Role of Incentive Regulation and Regulatory Independence2. The Folklore of Sorting Algorithms
3. Does adult education at upper secondary level influence annual wage earnings?
4. Strategic Policy Options to Improve Irrigation Water Allocation Efficiency: Analysis on Egypt and Morocco
5. Group cooperation, inclusion and disaffected pupils: some responses to informal learning in the music classroom
6. Education Responses to Climate Change and Quality: Two Parts of the Same Agenda?
7. Healthy state, worried workers: North Carolina in the world economy
8. Regional dynamics in mountain areas and the need for integrated policies
9. Neural Network Modelling of Constrained Spatial Interaction Flows
10. Demand Potential for Goat Meat in Southern States: Empirical Evidence from a Multi-State Goat Meat Consumer Survey