Figure 2: Genetic algorithms and randomness
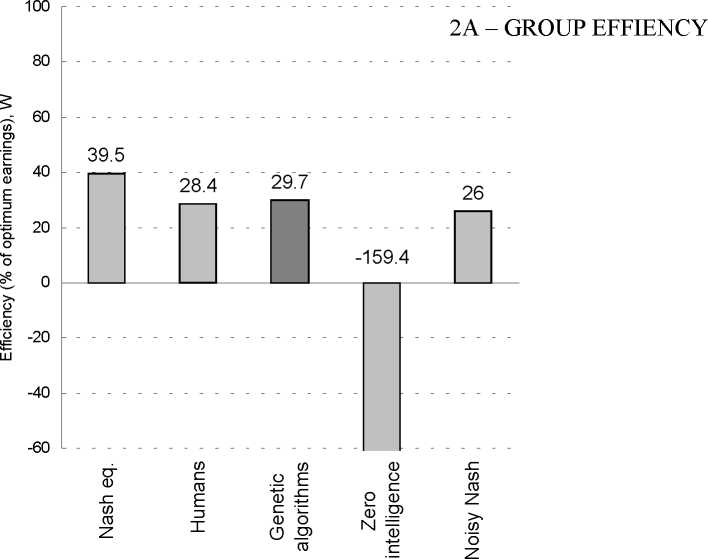
Notes: Nash equilibrium: prediction with selfish, perfectly rational agents; Human subjects: average of 4
experimental sessions; Genetic algorithm agents: selfish, boundedly rational agents (T=64,τ=32, average over 100
simulated runs, v.5.0); Zero-intelligence agents: random draws from a uniform distribution (average over 100
simulated runs v.5.6); xi ~U[0,θ] with xi iid, θ=50; Noisy Nash agents: are ZI with probability p and are best
responders to other Noisy Nash agents with probability (1-p).
More intriguing information
1. Connectionism, Analogicity and Mental Content2. The name is absent
3. The resources and strategies that 10-11 year old boys use to construct masculinities in the school setting
4. The name is absent
5. The name is absent
6. Conditions for learning: partnerships for engaging secondary pupils with contemporary art.
7. El Mercosur y la integración económica global
8. The name is absent
9. Yield curve analysis
10. Activation of s28-dependent transcription in Escherichia coli by the cyclic AMP receptor protein requires an unusual promoter organization