1
0.5
0
-0.5
-1

-1.2 -1 -0.8 -0.6 -0.4 -0.2 0 0.2 0.4 0.6 0.8
Fig. 4. Separation performed by the MLP-based network.
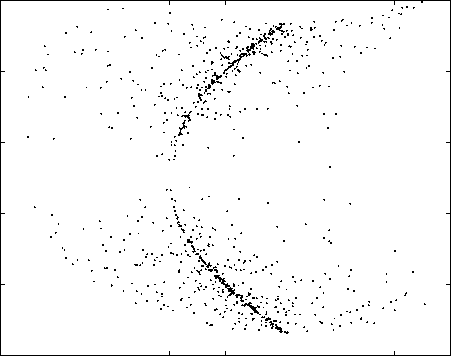
0.1
0.08
0.06
0.04
0.02
0
-0.02
-0.04
-0.06
-0.08
-0.1
-0.06 -0.04 -0.02 0 0.02 0.04 0.06 0.08 0.1
Fig. 6. Mixture of a supergaussian and a subgaussian source.
1.5
1
0.5
0
-0.5
-1
-1.5
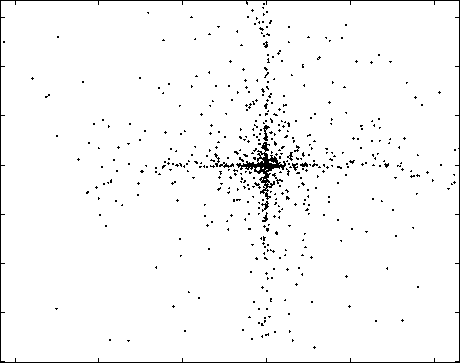
-1.5
-1
-0.5
0.5
Fig. 5. Separation performed by the RBF-based network.
1
-1
-1.5
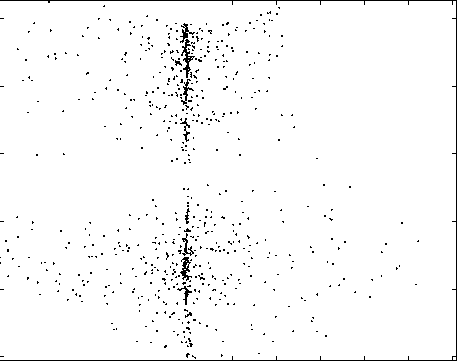
-0.4 -0.3 -0.2 -0.1 0 0.1 0.2 0.3 0.4 0.5 0.6
Fig. 7. Separation performed by the MLP-based network.
slow training of MLP-based nonlinear ICA systems is the
nonlocal character of these networks.
Figure 9 shows an example of an ICA result obtained
with the RBF-based network, in the case of the mixture of
two supergaussians, but without weight decay. While a rel-
atively good ICA result was achieved (the estimated mutual
information is the same as in Figs. 4 and 5), the original
sources were not separated. This shows the importance of
using regularization with networks of this kind.
5. CONCLUSIONS
We have briefly presented the MISEP, a method for linear
and nonlinear ICA, which is an extension of the well known
INFOMAX method. We discussed a possible cause for the
relatively slow learning that it sometimes shows, having
conjectured that it was due to the use of non-local units in
the network that performs the ICA operation.
This conjecture was confirmed by experimental tests, in
which a system based on a radial basis function network was
compared to one based on a multilayer perceptron on the
same nonlinear ICA problems. These tests confirmed that
that system based on RBF units learns significantly faster,
and shows a lower variability of the training times. The tests
also showed, however, that the RBF-based system needs to
have explicit regularization to be able to perform nonlin-
ear source separation, contrary to what happened with the
MLP-based one.
More intriguing information
1. Conflict and Uncertainty: A Dynamic Approach2. Shifting Identities and Blurring Boundaries: The Emergence of Third Space Professionals in UK Higher Education
3. Migration and Technological Change in Rural Households: Complements or Substitutes?
4. Cyclical Changes in Short-Run Earnings Mobility in Canada, 1982-1996
5. Studies on association of arbuscular mycorrhizal fungi with gluconacetobacter diazotrophicus and its effect on improvement of sorghum bicolor (L.)
6. The name is absent
7. The name is absent
8. How to do things without words: Infants, utterance-activity and distributed cognition.
9. Fiscal Reform and Monetary Union in West Africa
10. The name is absent