An impact- of the investment- constraint- on the
expected performance can be observed in more
detail in Figure 19. Two histograms of the dis-
counted total utility realisations arc presented.
The dark shadowed one corresponds to the un-
constrained policies (compare Figure 14). The
light- histogram represents the constrained pol-
icy performance. Evidently, the constrained policy
guarantees more “secure” performance (standard
deviation = 108 vis-à-vis 166 of the unconstrained
policy). However, the unconstrained policy brings
a (marginally) higher utility value. More compu-
tations of that kind would generate the “efficient-
boundary” .
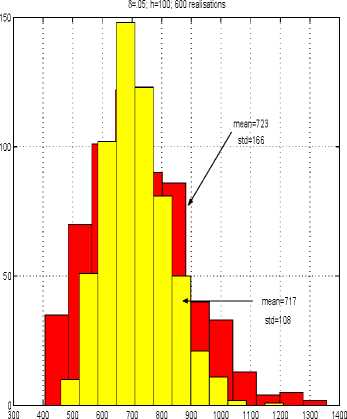
utility
Fig. 19. Utility realisation distributions.
In a similar way, another portfolio problem, in
which some minimal (or maximal) consumption
rate is given could be solved.
5.3 Pension funds
A practical problem of financial engineering is one
in which an agent- pays an amount- .τo to a pension
fund, to be repaid by a quantity xτ at time T. The
latter is a result- of an investment- policy uι(.τ)
adopted by the fund’s manager.
The manager’s policy depends on his or her ob-
jective function, which could be the maximisation
of an expected value, the minimisation of risk to
obtain a target- amount-, etc. Oncc the objective
function is revealed, the manager’s policy can
be computed as a solution to a stochastic opti-
mal control problem associated with the objec-
tive function. The problem solution will routinely
comprise an optimal decision rule uι(.τ), U2(x)
and a Monte-Carlo simulated distribution of xγ∙
Knowing the former is crucial for the manager to
control the portfolio. The latter is “practical” in
that it- tolls the pension buyer what they can, or
should, expect- as xτ-
Knowing the distribution of xγ also helps the
manager. It- gives them an idea of what prob-
abilities, or risks, arc associated with obtaining
particular realisations of the objective function.
For example, the distribution may suggest- that,
for every .tq there is a probable terminal value
xτ, which the manager may choose to advertise
as the pension target-
Wc will first- solve a pension fund problem for
the expected value criterion, as follows. In (4),
set- <z(X(t),u(t),t) = 0, s(T(t)) = .τ(T) 12
and suppose that the management- fee is 2%.τ(t).
This means that we need to solve an optimisation
problem in uɪ(æ) with U∙2(t) = .02.τ(t).
Using the Markovian approximation approach as
in Section 4.2, with the same model parameters
i.c., T = 10, r = .05, etc., generates a rather
trivial optimal strategy: t⅛ιt = l,U2,t = ∙02.τf for
positive states and times. Applying the strategy to
different- initial outlays .f,o generates the following
final fund yield spread and location measures13
at T = 10, see Figure 20.
The figure toll us, among other things, that an
initial deposit- of $40,000 corresponds to the ex-
pected pension value of about- $100,000. However,
the median fund’s yield for this objective function
is significantly below the mean. This indicates
that the fund distribution is skewed, which is evi-
dent- from Figure 21 (upper panel). The histogram
shows us too that the probability of earning less
than the “secure” revenue
40,000 cxp{(r — “management- fee") 10} = 53,994
is more than .5.14 It- is even fairly probable
(with probability >.4) to earn less than the initial
outlay .τo = 40,000. Evidently, using a policy
that maximises the expected yield is a very risky
strategy of managing a portfolio.
12Horo, the objective function is not HΛRΛ.
13Avoragod over 1000 realisations.
14To prove this and the subsequent claims integrate the
area under the histogram from zero to 53,994 and 40,000 ,
respectively.
13
More intriguing information
1. The name is absent2. Multifunctionality of Agriculture: An Inquiry Into the Complementarity Between Landscape Preservation and Food Security
3. The name is absent
4. Innovation Trajectories in Honduras’ Coffee Value Chain. Public and Private Influence on the Use of New Knowledge and Technology among Coffee Growers
5. The name is absent
6. ‘I’m so much more myself now, coming back to work’ - working class mothers, paid work and childcare.
7. The name is absent
8. Imperfect competition and congestion in the City
9. Comparative study of hatching rates of African catfish (Clarias gariepinus Burchell 1822) eggs on different substrates
10. Barriers and Limitations in the Development of Industrial Innovation in the Region